Summary
Today, leveraging analytics is no longer optional but necessary for organizations looking to gain a competitive edge. Within the broad data analytics umbrella, predictive analytics and prescriptive analytics have emerged as two of the most powerful tools in a business’s arsenal. However, confusion persists when comparing prescriptive vs predictive analytics, including their unique roles, benefits, and how they complement each other. This article provides clarity.
What is Predictive Analytics?
Predictive analytics focuses on what could occur based on observed trends, patterns, and behaviors from past and present data. It uses techniques such as data mining, statistical modeling, and AI to analyze historical and real-time data. The insights derived help businesses mitigate risks, predict churn, and provide personalized recommendations.
For example, Netflix employs predictive analytics to forecast what a subscriber will likely watch next based on their viewing history. Similarly, credit scoring—used for decades—relies on predictive models to estimate a borrower’s likelihood of repayment.
Key features of predictive analytics include:
- Forecasting potential future outcomes based on historical data.
- Relying on structured data like credit histories and transaction data.
- Providing consistent predictions based on the same dataset.
What is Prescriptive Analytics?
While predictive analytics forecasts future events, prescriptive analytics goes a step further. It identifies actionable steps that a person or organization can take in response to a forecast and evaluates the potential consequences of these actions.
Prescriptive analytics often leverages machine learning due to its need for large datasets. As new data becomes available, models continuously update to refine recommendations. This makes prescriptive analytics ideal for applications like risk management, optimization, and decision-making.
Key features of prescriptive analytics include:
- Delivering specific, actionable recommendations.
- Utilizing hybrid data (structured and unstructured).
- Continuously evolving models for up-to-date suggestions.
For instance, a company might use prescriptive analytics to optimize logistics by identifying the best shipping routes and schedules, ensuring cost savings and timely delivery.
Get advanced data insights for your domains with our big data analytics services and solutions.
Prescriptive vs Predictive Analytics Differences
Although prescriptive vs predictive analytics are interconnected, they serve distinct purposes and are not mutually exclusive. In reality, they are more like segments of the same, Namely, they are not completely separate processes in which one is more powerful or better than the other, but both are parts of a unified whole. Nevertheless, predictive analytics and prescriptive analysis do have some differences. Let’s examine the distinctions.
Feature | Predictive Analytics | Prescriptive Analytics |
---|---|---|
Focus | Forecasting future outcomes based on historical data | Providing actionable recommendations for optimal results |
Data Type | Structured (e.g., transaction history, customer data) | Hybrid (structured and unstructured) |
Model Update | Consistent predictions with the same data | Dynamic updates as new data becomes available |
Key Question Answered | What is likely to happen? | What should we do to achieve the best outcome? |
By understanding prescriptive vs predictive analytics, businesses can better utilize their data for forecasting and decision-making.
Predictive Analytics and Prescriptive Analytics Example
Predictive Analytics Example
In retail, predictive analytics can forecast future demand based on customer behavior and historical sales data. For instance, a retailer might predict a 20% increase in sales for a product during the holiday season, helping them prepare inventory accordingly.
Prescriptive Analytics Example
Building on the prediction, prescriptive analytics can recommend specific actions to meet the demand. It might suggest increasing inventory by 30%, optimizing delivery schedules, and allocating additional staff during peak hours. These insights enable businesses to boost operational efficiency and profitability.
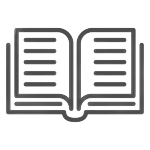
People Also Read
Conclusion
Both predictive analytics and prescriptive analytics play crucial roles in data-driven decision-making. When considering prescriptive vs predictive analytics, it’s clear that they are complementary rather than competing. Predictive analytics answers, “What is likely to happen?” by identifying trends and patterns, while prescriptive analytics addresses, “What should we do to achieve the best outcome?” Together, they empower businesses to anticipate challenges, seize opportunities, and make well-informed, profitable decisions.
By understanding the nuances of prescriptive vs predictive analytics and staying informed about the latest data analytics trends, organizations can maximize the value of their data analytics strategies.